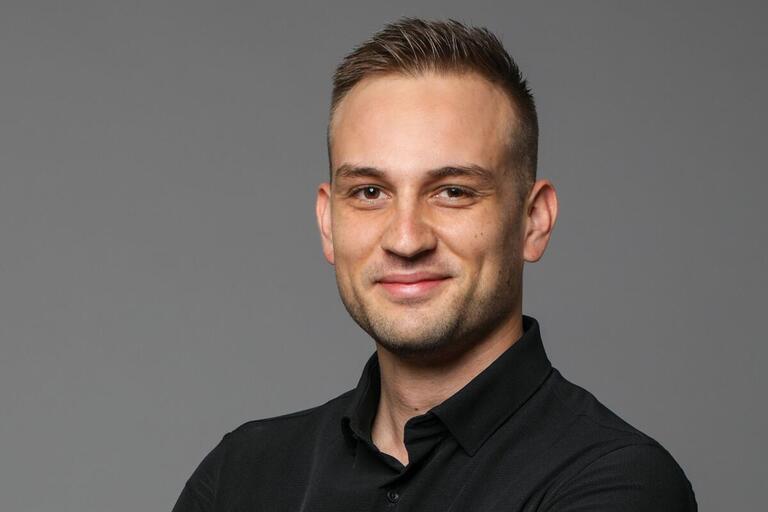
SEM217: Stjepan Begušić, University of Zagreb: Issues in large covariance matrix estimation for portfolio risk prediction
Tuesday, November 29th @ 11:00-12:30 PM (ONLINE) Meeting ID: 995 9778 2168
Most covariance matrix estimation studies are focused on portfolio optimization applications, ultimately mitigating the "error maximization" property of optimizers. However, correcting one kind of error might lead to introducing errors in other applications, such as portfolio risk measurement. This talk is focused on covariance estimation issues which arise in the application of risk prediction for different portfolios. Results for a range of dimensionality regimes and various portfolios will be discussed, together with some insights for practitioners and directions for future work.

SEM217: Jose Menchero, Bloomberg: Advances in Estimating Factor Correlations
Tuesday, September 6th @ 11:00-12:30 PM, RM 648 Evans Hall
Covariance matrices are used in finance for two basic purposes: predicting portfolio volatility and constructing optimal portfolios. Covariance matrices that work well for one use case may work poorly for the other use case, especially when the dimensionality is high. In this seminar, we present a technique for estimating large covariance matrices that produces reliable results for both volatility forecasting as well as portfolio optimization.
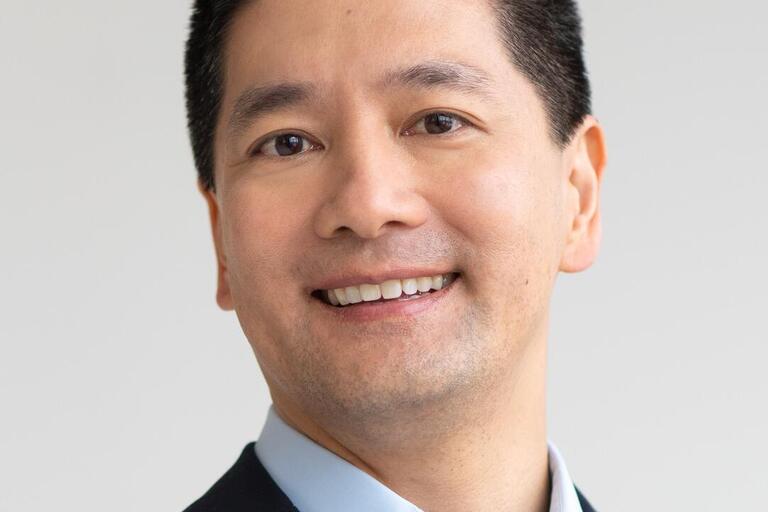
SEM217: Andrew Ang, BlackRock: Systematic Sustainable Alpha
Tuesday, September 13th @ 11:00-12:30 PM (RECORDING)
Andrew Ang, PhD, Managing Director, is Head of Factors, Sustainable and Solutions (FS-Squared). He also serves as Senior Advisor to BlackRock Retirement Solutions. As part of BlackRock Systematic, FS-squared is responsible for proprietary factor investing, delivering cutting-edge sustainable alpha, ESG outcomes and product innovation.
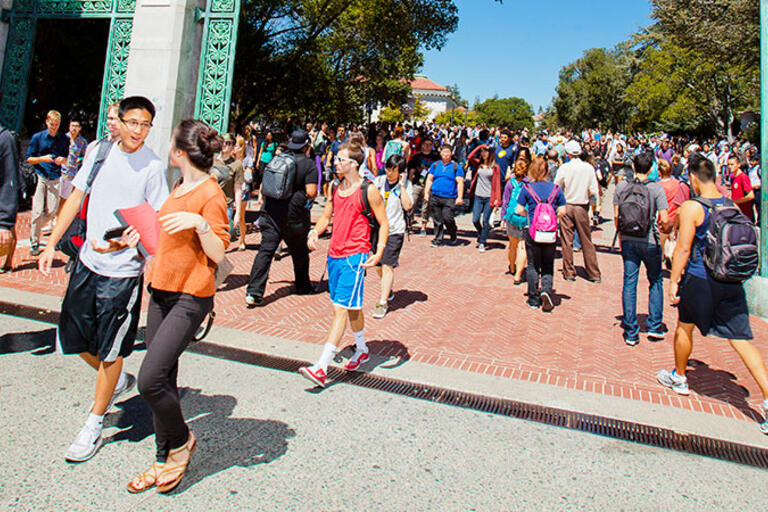
SEM217: Alex Lipton, Abu Dhabi Investment Authority: Forex Trading Utilizing Blockchains
Tuesday, September 20th @ 11:00-12:30 PM (ONLINE)
We argue that decentralized finance (DeFi) can be used to reorganize forex trading and market-making. Specifically, we show that an automated market-making (AMM) cross-settlement mechanism for digital assets on interoperable blockchains, handling central bank digital currencies (CBDCs) and stable coins, is a promising venue. We develop an innovative approach for generating fair exchange rates for on-chain assets consistent with traditional off-chain markets. Finally, we illustrate the efficacy of our approach on realized FX rates for G-10 currencies.
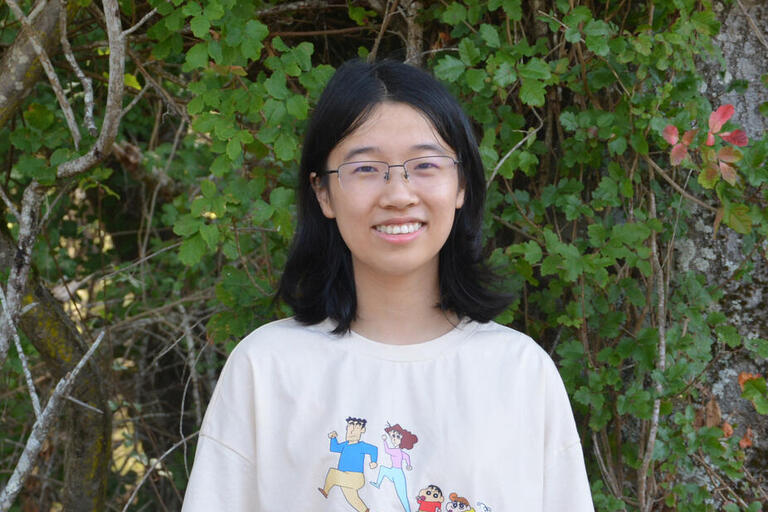
SEM217: Xinyi Zhong, Yale: Empirical Bayes PCA in high dimensions
Tuesday, September 27th @ 11:00-12:30 PM (RECORDING)
When the dimension of data is comparable to or larger than the number of data samples, Principal Components Analysis (PCA) may exhibit problematic high-dimensional noise. In this work, we propose an Empirical Bayes PCA method that reduces this noise by estimating a joint prior distribution for the principal components. EB-PCA is based on the classical Kiefer-Wolfowitz nonparametric MLE for empirical Bayes estimation, distributional results derived from random matrix theory for the sample PCs, and iterative refinement using an Approximate Message Passing (AMP) algorithm. In theoretical "spiked" models, EB-PCA achieves Bayes-optimal estimation accuracy in the same settings as an oracle Bayes AMP procedure that knows the true priors. Empirically, EB-PCA significantly improves over PCA when there is strong prior structure, both in simulation and on quantitative benchmarks constructed from the 1000 Genomes Project and the International HapMap Project. An illustration is presented for analysis of gene expression data obtained by single-cell RNA-seq.

SEM 217: Alec Kercheval, Florida State University: The James-Stein estimator for eigenvectors
Tuesday, October 4th @ 11:00-12:30 PM (RECORDING)
Portfolio risk forecasts require an estimate of the covariance matrix of asset returns, often for a large number of assets. When only a small number of observations are available, we are in the high-dimension-low-sample-size (HL) regime in which estimation error dominates. Factor models are used to decrease the dimension, but the factors still need to be estimated. We describe a shrinkage estimator for the first principal component, called James-Stein for Eigenvectors (JSE), that is parallel to the famous James-Stein estimator for a collection of averages. In the context of a 1-factor model, JSE substantially improves optimization-based metrics for the minimum variance portfolio. With certain extra information, JSE is a consistent estimator of the leading eigenvector. This is based on joint work with Lisa Goldberg, Hubeyb Gurdogan, and Alex Shkolnik.

SEM 217: Jeff Bohn, One Concern: Valuing and insuring natural assets: Challenges and opportunities
Tuesday, October 11th @ 11:00-12:30 PM, RM 648 Evans Hall (RECORDING)
As global population increases amidst rapid, continued urban development, lifeline networks (LN) (e.g., power, water, transportation, etc.) are becoming an important component of re/insurer’s catastrophic risk assessments. As climate change has increased the frequency or severity (and sometimes both) of natural disasters, a broader cross-section of financial firms is exploring how to incorporate resilience modeling into existing operational, physical, and credit risk models. Thus, more detailed catastrophic risk frameworks have become essential to assess and bolster the resilience (to low-frequency, high-severity natural disasters) of societies, properties, businesses, and governments. This growing material risk is not typically managed. In addition to these physical infrastructure LN, human-centered entities depend on eco-systems (e.g., clean air, clean water, productive soil, healthy oceans, healthy forests, etc.) These natural assets can be considered eco-systems services (ES). LN and ES together constitute material dependency risks. Generally, these networks and systems can be considered public goods and, in many cases, global public goods. As public goods, their value-generating capacity is typically non-rival and non-excludable making it harder to align incentives to invest and manage these resources—global public goods are particularly challenging. Herein lies a foundational challenge for managing natural assets.
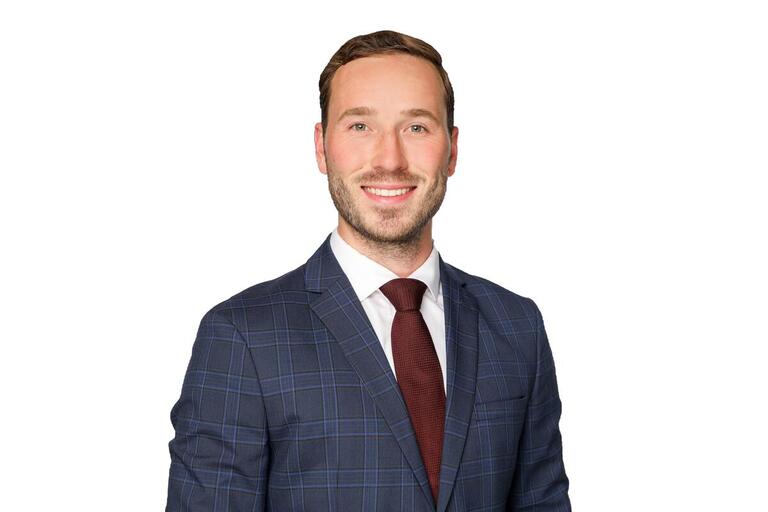
SEM217: Tizian Otto, University of Hamburg (visiting Stanford University): Estimating Stock Market Betas via Machine Learning
Tuesday, October 18th @ 11:00-12:30 PM
This paper evaluates the predictive performance of machine learning techniques in estimating time-varying market betas of U.S. stocks. Compared to established estimators, machine learning-based approaches outperform from both a statistical and an economic perspective. They provide the lowest forecast errors and lead to truly ex-post market-neutral portfolios. Among the different techniques, random forests perform the best overall. Moreover, the inherent model complexity is strongly time-varying. Historical betas, as well as turnover and size signals, are the most important predictors. Compared to linear regressions, interactions and nonlinear effects substantially enhance predictive performance.

SEM217: Lisa Goldberg, CDAR & Aperio by BlackRock: Is Index Concentration an Inevitable Consequence of Market-Capitalization Weighting?
Tuesday, November 1st @ 11:00-12:30 PM, RM 648 Evans Hall (ZOOM)
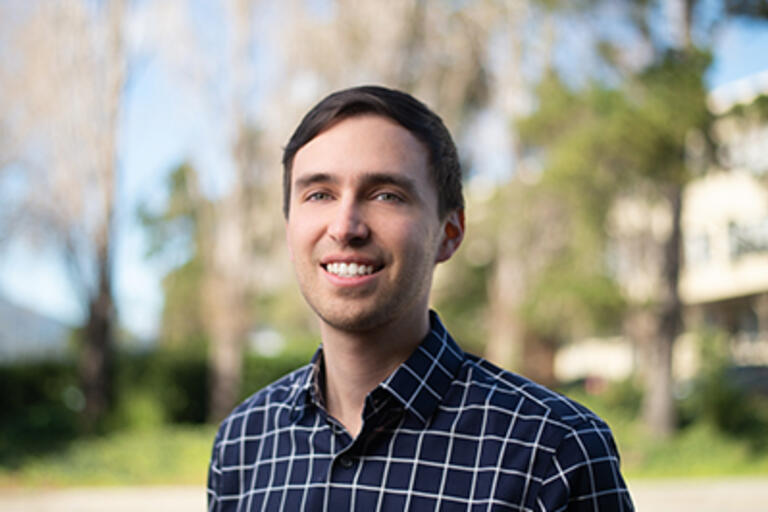
Harrison Selwitz, Aperio by BlackRock
Market-cap-weighted equity indexes are ubiquitous. However, there are growing concerns that such indexes are increasingly concentrated in a few stocks. We ask: Does market-cap weighting inevitably lead to increased concentration over time? The question of inevitability arises from research that suggests the possibility of dominance by a few firms over time via a variety of plausible causal mechanisms. We study concentration in major equity market indexes over time and show that, despite recent concerns, concentration is not yet at levels that may be problematic, and for some indexes was higher in the past. Monte Carlo simulations calibrated to market data provide insight into various approaches to slow concentration, albeit at the expense of higher turnover.
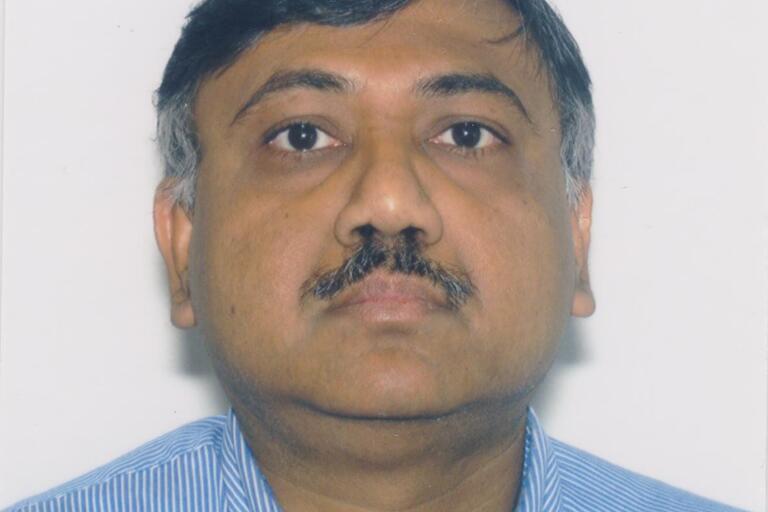
SEM 217: Debashis Paul, UC Davis: Regularized Testing of Linear Hypotheses in High Dimensions
Tuesday, November 8th @ 11:00-12:30 PM (ZOOM)
We consider the problem of testing linear hypotheses associated with a multivariate linear regression model. Classical tests for this type of hypotheses based on the likelihood ratio statistics suffer from substantial loss of power when the dimensionality of the observations is comparable to the sample size. To mitigate this problem, we propose two different classes of regularized test procedures that rely on a nonlinear shrinkage of the eigenvalues, and possibly eigenprojections, of the estimated noise covariance matrix. The first approach utilizes a ridge-type shrinkage, while the second works under the structural assumption that the population noise covariance matrix has a spiked eigenvalue structure. We address the problem of finding the optimal regularization parameter in each case by making use of decision-theoretic principles.

SEM217: Petter Kolm, NYU: Deep Order Flow Imbalance: Extracting Alpha at Multiple Horizons from the Limit Order Book
Tuesday, November 15th @ 11:00-12:30 PM (ZOOM)
We describe how deep learning methods can be applied to forecast stock returns from high frequency order book states. We review the literature in this area and describe a study where we evaluate return forecasts for several deep learning models for a large subset of symbols traded on the Nasdaq exchange. We investigate whether transformations of the order book states are necessary and relate the performance of deep learning models to the stocks' microstructural properties. In addition, we provide some color on hyperparameter sensitivity for the problem of high frequency return forecasting. This is joint work with Jeremy Turiel and Nicholas Westray.